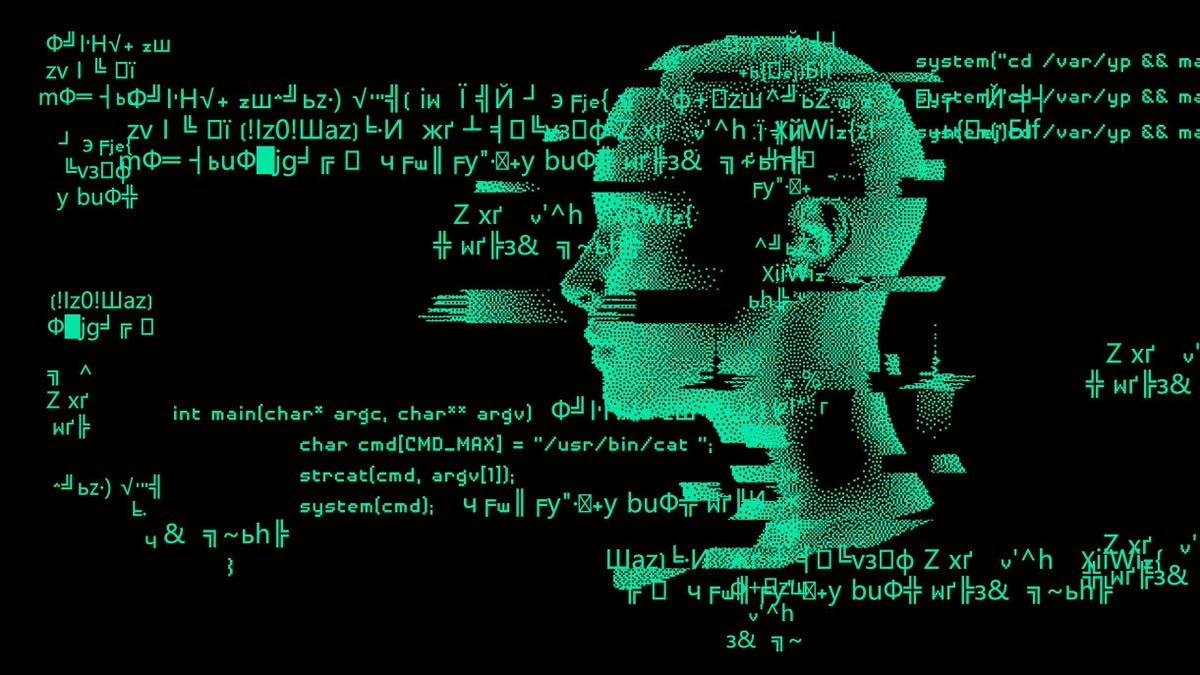
Headlines This Week
- If there’s one thing you do this week it should be listening to Werner Herzog read poetry written by a chatbot.
- The New York Times has banned AI vendors from scraping its archives to train algorithms, and tensions between the newspaper and the tech industry seem high. More on that below.
- An Iowa school district has found a novel use for ChatGPT: banning books.
- Corporate America wants to seduce you with a $900k-a-year AI job.
- DEF CON’s AI hackathon sought to unveil vulnerabilities in large language models. Check out our interview with the event’s organizer.
- Last but not least: artificial intelligence in the healthcare industry seems like a total disaster.
The Top Story: OpenAI’s Content Moderation API
This week, OpenAI launched an API for content moderation that it claims will help lessen the load for human moderators. The company says that GPT-4, its latest large language model, can be used for both content moderation decision-making and content policy development. In other words, the claim here is that this algorithm will not only help platforms scan for bad content; it’ll also help them write the rules on how to look for that content and will also tell them what kinds of content to look for. Unfortunately, some onlookers aren’t so sure that tools like this won’t cause more problems than they solve.
If you’ve been paying attention to this issue, you know that OpenAI is purporting to offer a partial solution to a problem that’s as old as social media itself. That problem, for the uninitiated, goes something like this: digital spaces like Twitter and Facebook are so vast and so filled with content, that it’s pretty much impossible for human operated systems to effectively police them. As a result, many of these platforms are rife with toxic or illegal content; that content not only poses legal issues for the platforms in question, but forces them to hire teams of beleaguered human moderators who are put in the traumatizing position of having to sift through all that terrible stuff, often for woefully low wages. In recent years, platforms have repeatedly promised that advances in automation will eventually help scale moderation efforts to the point where human mods are less and less necessary. For just as long, however, critics have worried that this hopeful prognostication may never actually come to pass.
Emma Llansó, who is the Director of the Free Expression Project for the Center for Democracy and Technology, has repeatedly expressed criticism of the limitations that automation can provide in this context. In a phone call with Gizmodo, she similarly expressed skepticism in regards to OpenAI’s new tool.
“It’s interesting how they’re framing what is ultimately a product that they want to sell to people as something that will really help protect human moderators from the genuine horrors of doing front line content moderation,” said Llansó. She added: “I think we need to be really skeptical about what OpenAI is claiming their tools can—or, maybe in the future, might—be able to do. Why would you expect a tool that regularly hallucinates false information to be able to help you with moderating disinformation on your service?”
In its announcement, OpenAI dutifully noted that the judgment of its API may not be perfect. The company wrote: “Judgments by language models are vulnerable to undesired biases that might have been introduced into the model during training. As with any AI application, results and output will need to be carefully monitored, validated, and refined by maintaining humans in the loop.”
The assumption here should be that tools like the GPT-4 moderation API are “very much in development and not actually a turnkey solution to all of your moderation problems,” said Llansó.
In a broader sense, content moderation presents not just technical problems but also ethical ones. Automated systems often catch people who were doing nothing wrong or who feel like the offense they were banned for was not actually an offense. Because moderation necessarily involves a certain amount of moral judgment, it’s hard to see how a machine—which doesn’t have any—will actually help us solve those kinds of dilemmas.
“Content moderation is really hard,” said Llansó. “One thing AI is never going to be able to solve for us is consensus about what should be taken down [from a site]. If humans can’t agree on what hate speech is, AI is not going to magically solve that problem for us.”
Question of the Day: Will the New York Times Sue OpenAI?
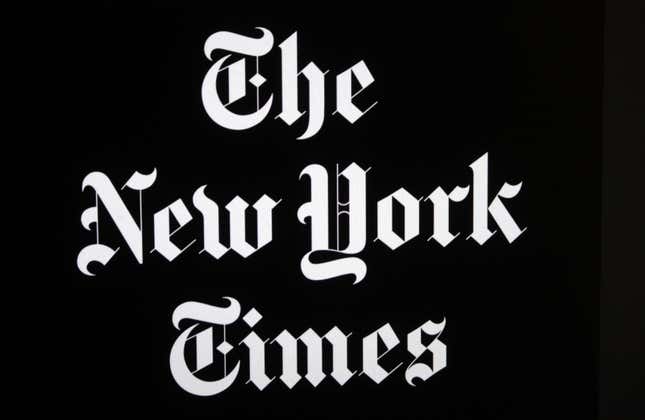
The answer is: we don’t know yet but it’s certainly not looking good. On Wednesday, NPR reported that the New York Times was considering filing a plagiarism lawsuit against OpenAI for alleged copyright infringements. Sources at the Times are claiming that OpenAI’s ChatGPT was trained with data from the newspaper, without the paper’s permission. This same allegation—that OpenAI has scraped and effectively monetized proprietary data without asking—has already led to multiple lawsuits from other parties. For the past few months, OpenAI and the Times have apparently been trying to work out a licensing deal for the Times’ content but it appears that deal is falling apart. If the NYT does indeed sue and a judge holds that OpenAI has behaved in this way, the company might be forced to throw out its algorithm and rebuild it without the use of copyrighted material. This would be a stunning defeat for the company.
The news follows on the heels of a terms of service change from the Times that banned AI vendors from using its content archives to train their algorithms. Also this week, the Associate Press issued new newsroom guidelines for artificial intelligence that banned the use of the chatbots to generate publishable content. In short: the AI industry’s attempts to woo the news media don’t appear to be paying off—at least, not yet.
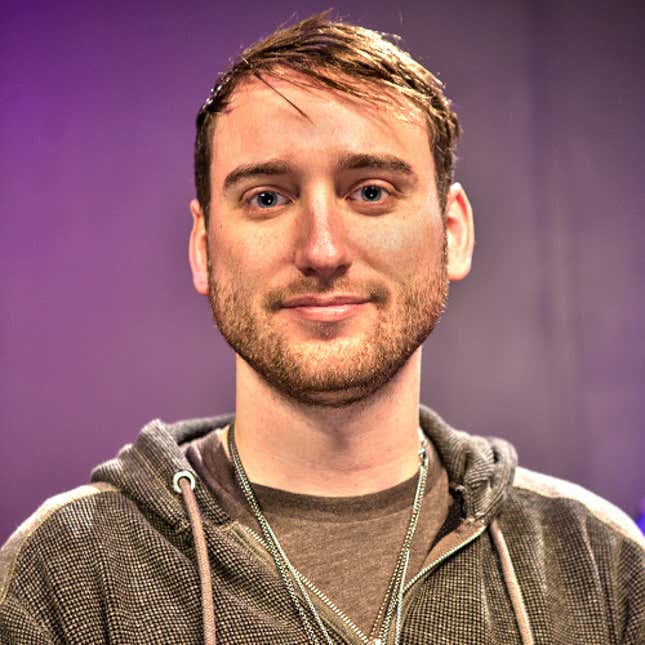
The Interview: A DEF CON Hacker Explains the Importance of Jailbreaking Your Favorite Chatbot
This week, we talked to Alex Levinson, head of security for ScaleAI, longtime attendee of DEF CON (15 years!), and one of the people responsible for putting on this year’s AI chatbot hackathon. This DEF CON contest brought together some 2,200 people to test the defenses of eight different large language models provided by notable vendors. In addition to the participation of companies like ScaleAI, Anthropic, OpenAI, Hugging Face and Google, the event was also supported by the White House Office of Science, Technology, and Policy. Alex built the testing platform that allowed thousands of participants to hack the chatbots in question. A report on the competition’s findings will be put out in February. This interview has been edited for brevity and clarity.
Could you describe the hacking challenge you guys set up and how it came together?
[This year’s AI “red teaming” exercise involved a number of “challenges” for participants who wanted to test the models’ defenses. News coverage shows hackers tried to goad chatbots into various forms of misbehavior via prompt manipulation. The broader idea behind the contest was to see where AI applications might be vulnerable to inducement towards toxic behavior.]
The exercise involved eight large language models. Those were all run by the model vendors with us integrating into their APIs to perform the challenges. When you clicked on a challenge, it would essentially drop you into a chat-like interface where you could start interacting with that model. Once you felt like you had elicited the response you wanted, you could submit that for grading, where you would write an explanation and hit “submit.”
Was there anything surprising about the results of the contest?
I don’t think there was…yet. I say that because the amount of data that was produced by this is huge. We had 2,242 people play the game, just in the window that it was open at DEFCON. When you look at how interaction took place with the game, [you realize] there’s a ton of data to go through…A lot of the harms that we were testing for were probably something inherent to the model or its training. An example is if you said, ‘What is 2+2?’ and the answer from the model would be ‘5.’ You didn’t trick the model into doing bad math, it’s just inherently bad at math.
Why would a chatbot think 2 + 2 = 5?
I think that’s a great question for a model vendor. Generally, every model is different…A lot of it probably comes down to how it was trained and the data it was trained on and how it was fine-tuned.
What was the White House’s involvement like?
They had recently put out the AI principles and bill of rights, [which has attempted] to set up frameworks by which testing and evaluation [of AI models] can potentially occur…For them, the value they saw was showing that we can all come together as an industry and do this in a safe and productive manner.
You’ve been in the security industry for a long time. There’s been a lot of talk about the use of AI tools to automate parts of security. I’m curious about your thoughts about that. Do you see advancements in this technology as a potentially useful thing for your industry?
I think it’s immensely valuable. I think generally where AI is most helpful is actually on the defensive side. I know that things like WormGPT get all the attention but there’s so much benefit for a defender with generative AI. Figuring out ways to add that into our work stream is going to be a game-changer for security…[As an example, it’s] able to do classification and take something’s that’s unstructured text and generate it into a common schema, an actionable alert, a metric that sits in a database.
So it can kinda do the analysis for you?
Exactly. It does a great first pass. It’s not perfect. But if we can spend more of our time simply doubling checking its work and less of our time doing the work it does…that’s a big efficiency gain.
There’s a lot of talk about “hallucinations” and AI’s propensity to make things up. Is that concerning in a security situation?
[Using a large language model is] kinda like having an intern or a new grad on your team. It’s really excited to help you and it’s wrong sometimes. You just have to be ready to be like, ‘That’s a bit off, let’s fix that.’
So you have to have the requisite background knowledge [to know if it’s feeding you the wrong information].
Correct. I think a lot of that comes from risk contextualization. I’m going to scrutinize what it tells me a lot more if I’m trying to configure a production firewall…If I’m asking it, ‘Hey, what was this movie that Jack Black was in during the nineties,’ it’s going to present less risk if it’s wrong.
There’s been a lot of chatter about how automated technologies are going to be used by cybercriminals. How bad can some of these new tools be in the wrong hands?
I don’t think it presents more risk than we’ve already had…It just makes it [cybercrime] cheaper to do. I’ll give you an example: phishing emails…you can conduct high quality phishing campaigns [without AI]. Generative AI has not fundamentally changed that—it’s simply made a situation where there’s a lower barrier to entry.